
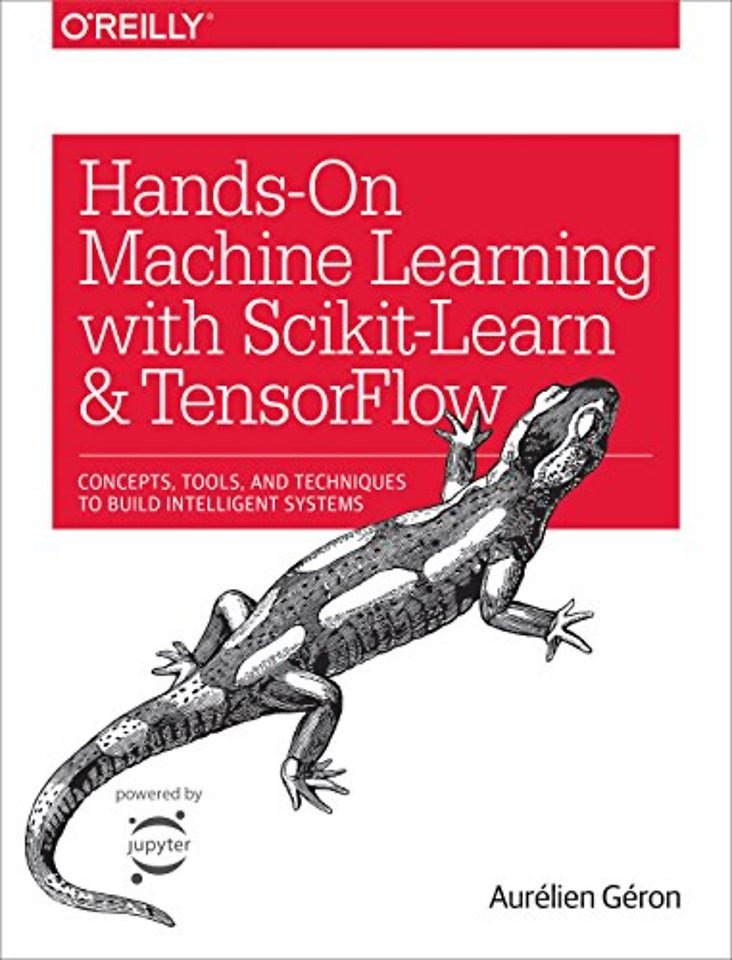
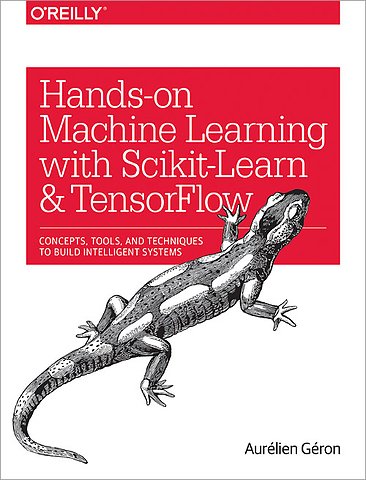
Aurélien Géron is a Machine Learning consultant. A former Googler, he led the YouTube video classification team from 2013 to 2016.
Meer over Aurélien GéronHands–On Machine Learning with Scikit–Learn and TensorFlow
Concepts, Tools, and Techniques to Build Intelligent Systems
Paperback Engels 2017 1e druk 9781491962299Samenvatting
Through a series of recent breakthroughs, deep learning has boosted the entire field of machine learning. Now, even programmers who know close to nothing about this technology can use simple, efficient tools to implement programs capable of learning from data. This practical book shows you how.
By using concrete examples, minimal theory, and two production-ready Python frameworks—scikit-learn and TensorFlow—author Aurélien Géron helps you gain an intuitive understanding of the concepts and tools for building intelligent systems. You’ll learn a range of techniques, starting with simple linear regression and progressing to deep neural networks. With exercises in each chapter to help you apply what you’ve learned, all you need is programming experience to get started.
- Explore the machine learning landscape, particularly neural nets
- Use scikit-learn to track an example machine-learning project end-to-end
- Explore several training models, including support vector machines, decision trees, random forests, and ensemble methods
- Use the TensorFlow library to build and train neural nets
- Dive into neural net architectures, including convolutional nets, recurrent nets, and deep reinforcement learning
- Learn techniques for training and scaling deep neural nets
- Apply practical code examples without acquiring excessive machine learning theory or algorithm details
Specificaties
Inhoudsopgave
Part 1: The Fundamentals of Machine Learning
1. The Machine Learning Landscape
What Is Machine Learning?
Why Use Machine Learning?
Types of Machine Learning Systems
Main Challenges of Machine Learning
Testing and Validating
Exercises
2. End-to-End Machine Learning Project
Working with Real Data
Look at the Big Picture
Get the Data
Discover and Visualize the Data to Gain Insights
Prepare the Data for Machine Learning Algorithms
Select and Train a Model
Fine-Tune Your Model
Launch, Monitor, and Maintain Your System
Try It Out!
Exercises
3. Classification
MNIST
Training a Binary Classifier
Performance Measures
Multiclass Classification
Error Analysis
Multilabel Classification
Multioutput Classification
Exercises
4. Training Models
Linear Regression
Gradient Descent
Polynomial Regression
Learning Curves
Regularized Linear Models
Logistic Regression
Exercises
5. Support Vector Machines
Linear SVM Classification
Nonlinear SVM Classification
SVM Regression
Under the Hood
Exercises
6. Decision Trees
Training and Visualizing a Decision Tree
Making Predictions
Estimating Class Probabilities
The CART Training Algorithm
Computational Complexity
Gini Impurity or Entropy?
Regularization Hyperparameters
Regression
Instability
Exercises
7. Ensemble Learning and Random Forests
Voting Classifiers
Bagging and Pasting
Random Patches and Random Subspaces
Random Forests
Boosting
Stacking
Exercises
8. Dimensionality Reduction
The Curse of Dimensionality
Main Approaches for Dimensionality Reduction
PCA
Kernel PCA
LLE
Other Dimensionality Reduction Techniques
Exercises
Part 2: Neural Networks and Deep Learning
9. Up and Running with TensorFlow
Installation
Creating Your First Graph and Running It in a Session
Managing Graphs
Lifecycle of a Node Value
Linear Regression with TensorFlow
Implementing Gradient Descent
Feeding Data to the Training Algorithm
Saving and Restoring Models
Visualizing the Graph and Training Curves Using TensorBoard
Name Scopes
Modularity
Sharing Variables
Exercises
10. Introduction to Artificial Neural Networks
From Biological to Artificial Neurons
Training an MLP with TensorFlow’s High-Level API
Training a DNN Using Plain TensorFlow
Fine-Tuning Neural Network Hyperparameters
Exercises
11. Training Deep Neural Nets
Vanishing/Exploding Gradients Problems
Reusing Pretrained Layers
Faster Optimizers
Avoiding Overfitting Through Regularization
Practical Guidelines
Exercises
12. Distributing TensorFlow Across Devices and Servers
Multiple Devices on a Single Machine
Multiple Devices Across Multiple Servers
Parallelizing Neural Networks on a TensorFlow Cluster
Exercises
13. Convolutional Neural Networks
The Architecture of the Visual Cortex
Convolutional Layer
Pooling Layer
CNN Architectures
Exercises
14, Recurrent Neural Networks
Recurrent Neurons
Basic RNNs in TensorFlow
Training RNNs
Deep RNNs
LSTM Cell
GRU Cell
Natural Language Processing
Exercises
15. Autoencoders
Efficient Data Representations
Performing PCA with an Undercomplete Linear Autoencoder
Stacked Autoencoders
Unsupervised Pretraining Using Stacked Autoencoders
Denoising Autoencoders
Sparse Autoencoders
Variational Autoencoders
Other Autoencoders
Exercises
16. Reinforcement Learning
Learning to Optimize Rewards
Policy Search
Introduction to OpenAI Gym
Neural Network Policies
Evaluating Actions: The Credit Assignment Problem
Policy Gradients
Markov Decision Processes
Temporal Difference Learning and Q-Learning
Learning to Play Ms. Pac-Man Using Deep Q-Learning
Exercises
Thank You!
Appendix A: Exercise Solutions
Appendix B: Machine Learning Project Checklist
Appendix C: SVM Dual Problem
Appendix D: Autodiff
Appendix E: Other Popular ANN Architectures
Index
Anderen die dit boek kochten, kochten ook
Rubrieken
- advisering
- algemeen management
- coaching en trainen
- communicatie en media
- economie
- financieel management
- inkoop en logistiek
- internet en social media
- it-management / ict
- juridisch
- leiderschap
- marketing
- mens en maatschappij
- non-profit
- ondernemen
- organisatiekunde
- personal finance
- personeelsmanagement
- persoonlijke effectiviteit
- projectmanagement
- psychologie
- reclame en verkoop
- strategisch management
- verandermanagement
- werk en loopbaan